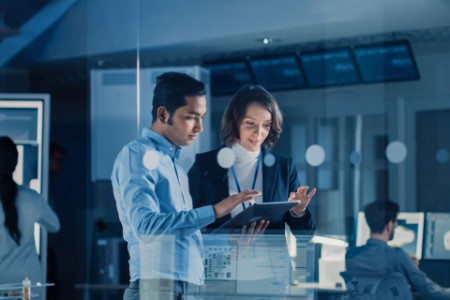
Chapter 1
To what extent can GenAI power productivity and economic growth?
The capabilities of GenAI result in a potential “virtuous cycle" of adoption, productivity acceleration and technological improvements.
GenAI has emerged as one of the most important technological revolutions in recent history and is considered a general-purpose technology (GPT), a concept first developed by Timothy Bresnahan and Manuel Trajtenberg in 1992.
GPT describes key technologies that can apply across a broad range of sectors and occupations and that have the capacity to improve over time and generate a wave of complementary innovations. For instance, the steam engine, electricity and the computer were important GPTs that fulfilled these criteria and were underpinned by a virtuous cycle of mutually reinforcing technological and economic forces.1
“The virtuous cycle of innovation spurred by general-purpose technologies can have a substantial effect on the economy,” observes Lydia Boussour, EY-Parthenon Senior Economist, Strategy and Transactions, Ernst & Young LLP.
For instance, David Byrne, Stephen Oliner and Daniel Sichel estimate that the information technology (IT) sector, which represents only a small share of the economy (less than 5% in the late 1990s), accounted for half of labor productivity growth in the US nonfarm sector between 1974 and 2004.2
The virtuous cycle of innovation spurred by general-purpose technologies can have a substantial effect on the economy.
And while productivity growth has slowed in recent decades, the contribution from the IT sector to US productivity growth has remained sizable, accounting for more than a third of the growth in labor productivity between 2004 and 2012.
Looking back at the emergence of the computer age, it began with large and expensive electronic computers that lacked adaptability and were designed for a few specific applications such as military purposes. The world’s first general-purpose electronic computer — the Electronic Numerical Integrator and Computer (ENIAC) — was developed in the mid-1940s to calculate artillery firing tables for the United States Army's Ballistic Research Laboratory.
Over time, though, improvements in computer processing performance and decreasing costs allowed more industries to adopt computers and enabled new innovations as well that, in turn, led to even wider computer adoption. This continued process of IT innovation and development and increased demand eventually gave way to a period of sustained gains in productivity and living standards.
Based on the level and speed of 1990s IT-driven acceleration in productivity growth, we estimate that GenAI has the potential to lift productivity growth by 50% to 100% in the coming decade. However, it will likely fall short of the doubling or tripling of productivity growth resulting from the Industrial Revolution or electricity adoption.
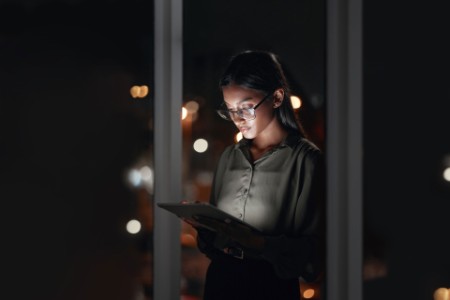
Chapter 2
Why the productivity boost from GenAI could lag
Time needed for GenAI adoption, skill development and “right sizing" applications will introduce lags to the diffusion of GenAI’s economic benefits.
The emergence of important technological innovations tends to have a limited initial effect on productivity and economic growth. During previous eras of technological progress, there has generally been a long delay between the inception of paradigm-shifting technologies and their diffusion across the economy and society.
The First Industrial Revolution, which took place in the mid-18th century in Great Britain, was a period of unparalleled progress marked by the birth of the steam engine, pioneered by James Watt, which transformed mining and transportation but also unleashed a wave of technological innovations (e.g., rollers, spinning and weaving machines, steam locomotives) that revolutionized numerous industries.
Although the steam engine represented an important technological breakthrough, it was only about 80 years later — in the second half of the 19th century — that it delivered a boost to aggregate productivity and raised economic prosperity.3 This occurred only when the steam technology improved and was adopted more broadly as a productivity-enhancing technology.
Living standards in Great Britain experienced slow growth in the early years of Britain’s Industrial Revolution. Real GDP per capita only grew at a compounded annual rate of 0.4% between 1750 and 1800. Eventually, however, the Industrial Revolution led to a large increase in living standards with GDP per capita doubling by 1870 and growing nearly two times faster at a compounded annual rate of about 0.75% from 1800 to 1900.
A similar productivity delay occurred with electricity, though the observed lag was shorter than for the steam engine. While the electrical age began in the 1880s in the US, it was only in the 1920s when electrification made critical advances that led to a sizable boost to productivity.4
The paradox of rapid technological progress alongside subdued productivity was also a feature of the IT revolution, which began in the 1970s with the introduction of the personal computer and the internet. In 1987, Robert Solow, the Nobel Prize economist, famously observed that “you can see the computer age everywhere but in the productivity statistics.”
At the time, growth in labor productivity as measured by real output per hour had slowed to a disappointing 0.5% annual pace despite major technological advances. It was only two decades later in the late 1990s and early 2000s that the paradox was resolved when labor productivity consistently surpassed 2% per year between 1998 and 2005 as IT technologies diffused more broadly throughout the economy.5
Faster speed of diffusion and adoption
We find that while companies are investing in AI — 43% of CEOs have already begun investing and another 45% plan to do so in the next year, according to the EY CEO Outlook Pulse survey — many are pursuing quick efficiency gains rather than more fundamental changes to maximize AI’s growth potential. And 90% of organizations are still in the earliest stages of AI maturity.
There are various potential sources of delay between a technological revolution and its boost to productivity, but three are critical:
- Implementation and diffusion: it takes time for new technologies to be adopted and diffused throughout an economy. Even after a technology is introduced, businesses might delay the adoption due to high up-front costs, uncertainty about its benefits or simply because they are waiting to see if even better technologies emerge.
- Learning and adjustment period: once technology is adopted, there is a period during which workers and managers must learn how to use it effectively: this involves trial and error, training and the development of leading practices.
- Complementary innovations: some technologies require complementary innovations or infrastructures to be fully effective.6 For instance, electric engines required a widespread electrical grid, and the benefits of personal computers were magnified once the internet became widespread.
The notable capabilities and performance of emerging AI tools already represent a big improvement over those introduced a decade ago such as cell phone virtual assistants.
While the broad-based productivity boost will likely occur with a lag, the speed of technological adoption and diffusion has increased from multiple decades in the 1800s to around 10 years in the computer age.
Faster diffusion and adoption of GenAI could mean the boost to economic activity will be felt in the next three to five years.
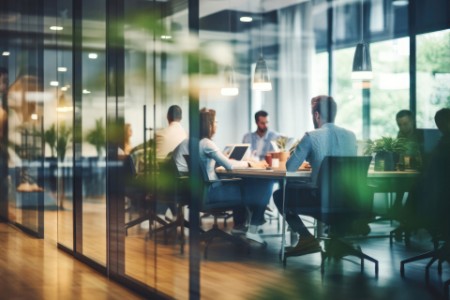
Chapter 3
Are fears about AI-driven mass unemployment warranted?
GenAI will likely eliminate specific tasks rather than occupations, as well as result in new jobs.
Technology has been reshaping the nature and organization of work for centuries, displacing some jobs while creating others, but apprehension about mass unemployment driven by technology has never been realized.
Today is not the first time that technological progress has sparked concerns that machines will render labor of people obsolete. In the late 16th century, Queen Elizabeth I refused to grant an English inventor named William Lee a patent for a mechanical knitting machine for fear it would displace manual labor.
Recently, these same fears have resonated with public opinion and in policy discussion given the rapid development of GenAI and its potential to automate tasks and jobs across many industries. Despite these concerns, the level of employment has continuously increased over the past century as new technologies ended up creating more jobs than they destroyed.
Impact of AI on labor
Technological innovations can affect labor in three main ways:
- Job creation: new technologies can lead to the emergence of new industries and sectors, creating new job opportunities. For instance, the rise of the internet brought about a range of jobs in web design, digital marketing, e-commerce and IT support that didn't exist before.
- Job displacement: conversely, automation and certain technological advancements can render some jobs obsolete. Routine and repetitive tasks, whether they are manual (like assembly line work) or cognitive (like data entry), are particularly susceptible to automation.
- Job transformation: many jobs are also transformed rather than eliminated by technology. This means that while some tasks within a job might be automated, other new tasks might emerge, or the nature of the job might change. For instance, bank tellers now do less cash handling due to ATMs but engage more in customer service roles.
The rapid mechanization of US agriculture in the early 20th century spurred one of the greatest structural shifts in employment in modern history. The introduction of new tools and machines led to a dramatic increase in productivity and a decrease in the demand for farm labor. Many displaced workers were forced to reskill for alternative employment such as factory work. This migration, which also responded to the labor demand created by the Industrial Revolution, led to a major reallocation in the US away from agriculture into non-agricultural occupations with the share of agriculture in overall employment falling from 55% in 1850 to 41% in 1900 and only 10% by 1950.
More recently, the digitization of the economy and automation have displaced and transformed many jobs by replacing some routine tasks that were once performed by the workforce — mostly low- and middle-skilled roles. But the information age has also led to rapid growth in IT-related employment with the creation of many new positions that never existed before such as data scientist, software engineer or web developer.
Between 1990 and 2001, US manufacturing employment declined by 12% while employment in computer systems design and related services tripled. Workers in the IT sector have also exhibited higher pay than the average worker in the private sector. Average hourly wages for workers in computer system design were 1.8 times higher than the average worker in 1990 and about double by the end of the 2000s.7
Because GenAI technologies are capable of performing some non-routine cognitive tasks, they have the potential to significantly alter the nature and content of jobs, especially for white-collar workers. But GenAI will likely displace specific tasks rather than entire occupations and will lead to the creation of new jobs such as AI trainers, ethicists or developers.
Strategic questions for business leaders to ask about the economic impact of AI
The macroeconomic ramifications of GenAI present an intricate tapestry for business leaders to unravel. Understanding the broader economic implications, while helping ensure the alignment of corporate strategy and ethics, becomes paramount. By navigating the following questions, business leaders can position their companies at the intersection of technological innovation and macroeconomic resilience, helping ensure sustainable growth in a world increasingly influenced by GenAI.
- Macroeconomic landscape awareness: how is leadership appraising and adapting to the changing macroeconomic landscape influenced by GenAI? Are there dedicated teams or individuals analyzing global economic trends, shifts in trade dynamics or potential disruptions in global supply chains resulting from AI breakthroughs?
- Innovation and economic growth: how is the company harnessing the power of GenAI to foster innovation that drives broader economic growth? Is the company exploring partnerships, collaborations or ecosystem engagements that amplify its economic impact through AI-driven innovations?
- Competitive landscape and strategic positioning: as GenAI reshapes industry structures and competitive dynamics, how is the company anticipating and navigating potential shifts in its industry's value chain? How are strategic investments in GenAI helping ensure the company's competitive edge in this evolving macroeconomic scenario?
- Economic externalities and value creation: how is leadership helping ensure that the company's GenAI strategy is generating sustainable economic value without inadvertently creating negative economic externalities? What measures are in place to evaluate the broader societal and economic impacts of the company's AI initiatives?
- Economic risk management: in the face of potential economic downturns or market uncertainties, how is the company leveraging GenAI for robust economic risk assessment and mitigation strategies? How are AI-driven predictive analytics aiding in proactive risk management?
- Labor market dynamics and talent strategy: with GenAI potentially disrupting labor markets, how is leadership ensuring that the company's talent strategy is both future-proof and ethically sound? Are there provisions for reskilling and upskilling employees to align with the changing landscape?
- Stakeholder engagement in a GenAI economy: how is the company engaging with its stakeholders — from investors to consumers — in articulating its value proposition in this GenAI-driven economic context? How are stakeholder perceptions and concerns being integrated into strategic decision-making?
Summary
This is the first installment of the EY-Parthenon macroeconomic article series on the economic impact of AI. The series aims to provide insights on the economic potential of generative AI, including new developments and actionable insights to arm companies’ decision-makers. The first article in this series covers learnings from three key historical episodes of rapid technological change that may help predict the economic impact of AI. Additional articles in the series will be published in the coming months.