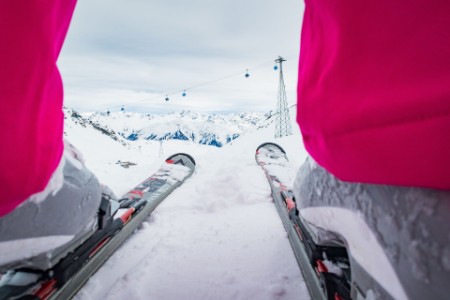
Chapter 1
Understand what AI technologies are capable of today
Some history: AGI or ANI?
The Dartmouth Conference held in 1956 kicked off a golden age of intense research into AI with the aim “of making a machine behave in ways that would be called intelligent if a human were so behaving.”2
Buoyed by impressive advances in the early days, AI pioneers like Marvin Minsky made bold claims that, “Within our lifetime machines may surpass us in general intelligence.” However, limited and expensive computing power and storage as well as a paucity of data meant that early solutions could only solve rudimentary problems. These technology limitations led to the first AI Winter where funding dried up and interest dwindled. The second AI Winter of 1980-87 was precipitated when expert systems became expensive to maintain and proved brittle when faced with unusual scenarios.
Perhaps even more detrimental was underestimating the difficulty of creating human-like or Artificial General Intelligence (AGI).
The current AI renaissance stems primarily from overcoming the technological hurdles that plagued earlier efforts. However, AI specialists today are not making proclamations of attaining AGI. Despite significant breakthroughs, Oren Etzioni, professor at the University of Washington and CEO of the Allen Institute for AI says, “We’re so far away from…even six-year-old level of intelligence, let alone full general human intelligence…”4
Artificial Narrow Intelligence (ANI)
While AGI may remain a long-term goal for some in the field, the current focus and enthusiasm is around Artificial Narrow Intelligence (ANI).
Cheap and abundant computer power, copious digital data generated by the proliferation of the internet, as well as Geoffrey Hinton’s breakthrough with deep learning, has led to an explosion of ANI applications. These applications execute single specific tasks in a limited context very well, sometimes better than humans. Today ANI algorithms are creating human value, powering digital voice assistants, driving product recommendations and aiding in cancer detection. They have also expanded human knowledge by finding new planets and deriving insights from human genetic data. The sheer number and diversity of commercial ANI applications is perhaps what sets this third wave of AI optimism apart.
With these accomplishments under its belt, has the eternal “spring” sprung for ANI?
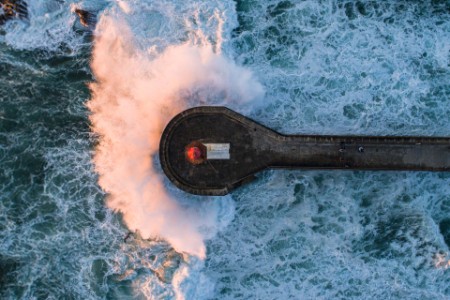
Chapter 2
Understand the price of a mistake
Your ANI is blooming. But is it infected with a costly human bias?
Current AI technologies can be applied to a wide spectrum of problems, each with different risk profiles. Care should be taken in matching the use case and the context with the appropriate technology.
ANI systems have become popular with companies, governments and entrepreneurs who are faced with a growing corpus of digital data waiting to be exploited. However, in pursuing ANI’s productivity and efficiency benefits, these stakeholders must consider the risks stemming from ANI’s shortcomings and the potential for unintentional human cost.
The most common criticisms of ANI include the algorithm’s inability to reason beyond its training data and it’s propensity to propagate inherent human biases as it learns from human generated data. While no technology is devoid of flaws, the cost of an error stemming from ANI’s drawbacks can have serious consequences especially in situations where the algorithm’s decision can substantially influence an individual’s fate.
In some cases, algorithmic errors are at worst inconvenient. For example, although digital voice assistants have made a faux pas or two resulting in awkward or unsettling moments for users, adoption and usage continues to soar. On the other hand, in high-profile public-facing contexts, algorithmic errors had catastrophic results and eroded the public’s trust. For example, recent fatalities involving self-driving cars dampened enthusiasm and led to a significant erosion of consumer confidence – a study conducted in 2018 found that 73% of US drivers would not trust a fully autonomous vehicle, compared to 63% in 2017.
As ANI-driven decision-making finds its way into other critical domains such as criminal justice, education and job recruitment, the price of a mistake has resulted in false arrests, racial bias, and gender discrimination. If the incidence of such errors increases, it could ultimately lead to a loss of trust in the technology entirely and leave this class of ANI applications vulnerable to a potential “winter”.
This is not to suggest that the entire field of ANI will falter. As Stefan Heck, co-founder and CEO of Nauto and EYQ Fellow suggests, “Perhaps we need another category between ANI and AGI to account for circumstances where failures could result in societal backlash.”
Definitions of AI and its various flavors have traditionally centered on the technology’s capability to mimic or surpass human physical and cognitive capabilities. While this framework has served to benchmark the technology’s evolution, it does not adequately reflect the risk profiles of algorithms when applied in different contexts.
How risky is your AI?
The framework below offers businesses and governments a way to categorize and classify their current and future AI applications.
Artificial Narrow Intelligence – Transactional (ANI-T) |
Artificial Narrow Intelligence – Consequential (ANI-C) |
Artificial General Intelligence (AGI) |
Artificial (ASI) |
|
---|---|---|---|---|
Definition | Single task in limited context as good as or better than human | Single task in dynamic context as good as or better than human | Multiple tasks across dynamic contexts as good as human | Surpass all human intellectual capabilities across known and unknown contexts |
Scope of Impact | Limited and short-term | Broad and long-term | Everything! | Unfathomable! |
Example | Digital voice assistants |
Autonomous cars | HAL 9000 | Beyond human imagination |
Risk Profile | Low | High | Unknown | Unknown |
Source: Stefan Heck & EYQ
Using this rubric business leaders and governments can assess the risk profile of their ANI-based use cases, strategy and investments. Addressing the risks, particularly with ANI-C applications, will not only be critical to the success of digital transformation efforts but also to maintaining the credibility and trust of businesses and governments with their customers and citizens. And consequently, it will allow them to realize the significant potential ANI holds to improve productivity, efficiency and the overall quality of life.
A variety of initiatives are underway to overcome the technological limitations of ANI and to mitigate the unwelcome consequences of algorithmic errors: new algorithmic approaches, frameworks for “Ethical AI” and the availability of open source tools to audit algorithms for bias, to name a few. The C-suite needs to take an active role in these initiatives and along with governments work to develop more ethical, equitable, accurate and transparent algorithms. Building trust in the technology will be essential to thwarting the risk of an impending “winter” for ANI-C applications.
Ultimately success will depend on business leaders and governments keeping human interests and human values central to the development of all forms of ANI solutions and minimizing or nullifying the price of an algorithmic mistake that could have far-reaching consequences for commercial performance or human welfare.

Chapter 3
Curb your enthusiasm: it’s no human brain (yet)
The myth, the expectations and the reality
Maybe our expectations of an AI spring are too high.
Cultural artefacts from Pygmalion to Frankenstein have consciously or subconsciously built humanity’s notion of what constitutes AI – an artificial being replete with the sentience, emotions, intellect and behaviors associated with humans.
Any technological breakthrough that implies a step towards realizing this vision of AI gets amplified and the potential for flaws are not factored into the public’s expectations. When a critical mistake ensues, hopes are dashed and the technology is deemed untrustworthy. In reality, AI technology today is not robust enough to cede decision-making in consequential human contexts. As Ray Edwards, GM ICT Business Development and Venture Capitalist at Yamaha Ventures, suggests “Some use cases will continue to require substantial human interaction and judgment before they can be commercially deployed at scale.”
Bridging the gap between our expectations and reality will be critical to thwarting another “winter” for any flavor of ANI (ANI-T or ANI-C). However, as Nigel Duffy, Global Innovation Artificial Intelligence Leader at EY, observes, “Aligning our expectations with reality has not occurred in the past and there is no certainty that we will be able to do it now. So the risk of another ‘AI Winter’ remains high”.
Holding back “winter”
For business leaders and governments with significant investments in ANI, minimizing the risk of a “winter” will involve:
- Engaging in a more balanced public discourse
- Acknowledging ANI’s flaws
- Managing their customers’ expectations.
- Thoughtfully developing and deploying ANI-C applications to promote trustworthy, equitable and ethical results.
Even with its current drawbacks, ANI holds great potential to improve the quality of life today. By projecting unrealistic expectations on ANI, we may be prevented from realizing its benefits.
As Roy Amara, former President of the Institute for the Future, observed: “We tend to overestimate the effect of a technology in the short run and underestimate the effect in the long run.” Even though ANI may not manifest in AGI in the near-term, a key imperative for business leaders and governments is to appropriately and safely leverage ANI, leading to new innovations that would deliver untold human value in the decades to come.
Summary
The most powerful step in negating future “AI Winters” may be harmonizing our expectations with the reality of where AI capabilities are today.