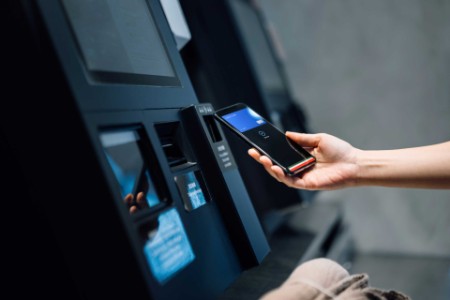
Chapter 1
Building trust in data
In today’s dynamic business environment, the lack of trustworthy data will inevitably act as a brake on digital transformation efforts.
Our EY Tech Horizon report, published in 2020, noted that aside from organisational issues, the single biggest reason that businesses struggle with transformation is because they overlook the fundamental fact that trustworthy data is a critical element for success.⁴ In a world where businesses are deluged with data from every customer click and keystroke, camera and connected sensor, and every supplier and employee, little wonder that quality, provenance, and lineage of data – all of which underpin trust – are seen as vital. Inaccurate or incomplete data can lead to mistakes ranging from the trivial, such as recommending the wrong product, to the highly damaging, including refusing a loan for an eligible customer. Mistakes such as these can sow the seeds of mistrust and diminish confidence in your data.
AI technology works well as far as there are defined data sets and there is accuracy in the data.
Over 40% of respondents in our survey for DCMS selected quality as the most important characteristic of their data. It was deemed to contribute most to success by organisations of varying size, age, sector and location. It should be no surprise, therefore, that 90% of respondents also indicated that they have a dedicated data strategy or data-improvement initiatives in place.
Figure 1. Most important data characteristics identified by survey respondents
Source: EY/DCMS
If nothing else, the downstream costs caused by data quality issues (including duplicated effort, process inefficiencies, potentially wasted future spending and other opportunity costs) can be eye-watering. Some estimates put the cost of remediating a data quality error at 10 times the cost of preventing it in the first place, and, by the time bad data causes strategic decisions to fail, the cost can balloon to 100 times.⁵
No leader knowingly uses poor quality data. They may assume the data they’re basing critical decisions on is to be trusted. But is it?
Despite many organisations already working on data quality initiatives, we also found some stakeholders who were not fully aware of how their data was being used, especially in relation to their adoption of AI. With many modern analytical tools and AI platforms making it easy to process data, with little or no understanding needed of the underlying technology, some organisations may be unaware of the consequences that even moderately poor data quality can have for business decision-making – let alone the potential implications for reputational risk or regulatory compliance breaches.
What can businesses do to build greater trust in their data?
For most businesses, data is the route to better decision-making. Data supports business goals, which are typically not defined in a way that specifies how, or whether, data is used to achieve them. In this context, data is merely an input – a set of facts or a stream of numbers that need to be marshalled into useful patterns. This implies that the relative importance of the different characteristics of data, such as quality, must be balanced against the impact of decision to be made.
In How to harness the power of trust and data, EY Global Chief Data Officer, Marco Vernocchi, suggests assessing risks across the dimensions of:⁶
- Strategic: the impact on your reputation, business potential, societal influence and overall competitiveness
- Operational: the impact on how you run your organisation, business processes, technologies and data
- Financial: the impact on your business performance, as well as current and future value
- Regulatory: the impact on compliance with standards, policies and laws
Related article
A robust data governance approach is, therefore, fundamental to the success of any data or AI programme. Data governance needs to be based on scientific processes, rather than just human judgement, and supported by appropriate technology.⁷ Such governance needs to consider whether your data is:
- Verified and accurate
- Immutable and free of bias
- Relevant and contextually complete for the types of decision being made
- Stored, used and reused in compliance with all laws and regulations
To build trust in the quality of data and how it is used across enterprise systems to support decision-making, many businesses are investing in a ‘data fabric’: a set of independent software services – functionality that can be used for a specific purpose, together with policies that control its use – to create a consistent and expanded data experience across the enterprise.⁸
These services use modern methods from the fields of AI and software engineering to provide capabilities such as data management and monitoring. They can:
- Track data throughout its life (ensuring its secure ingestion, processing and use)
- Reduce excessive movement, copying, and inconsistent use of data
- Eliminate duplication
- Implement data quality and control protocols (ensuring that all data and decisions they support can be trusted)
All services in the data fabric remain connected via an infrastructure layer called a ‘service mesh’, which provides secure, fast, fault-tolerant inter-service communication, along with other crucial functions such as load-balancing, encryption, tracing and monitoring. Collectively, the data fabric and service mesh provide greater resiliency and repeatability, which enables longer-term business planning and success.
Related article
Additionally, the data fabric’s services are orchestrated using a digital data catalogue, which links business metadata with technical metadata. This helps organisations manage the portfolio of applications that use data, by documenting changes in underlying data structures and incorporating specific policies and rules.
Typically, a data fabric includes a digital interface for users to access, execute and monitor each of these services. The interface allows the data office to view relevant operational and management information and dashboards, showing insights and performance.
A data fabric approach enables businesses to bring data to where it is needed most and to manage it reliably, while simplifying the implementation of consistent policies across the wider ecosystem of software applications and users who rely on the data for decision-making. The key benefits of a data fabric are:
- More datasets and platforms can be added easily (for example, in the case of mergers and acquisitions) by simply connecting new resources – external or internal.
- New services can be added or removed as needed, without disruption.
- It is technology- and platform-agnostic, which means that users accessing data services are abstracted from how the newer platforms are integrated to existing ones.
Fundamentally, a data fabric harmonises the different elements of a business, which would otherwise typically remain uncoordinated. This improves data quality, lineage and traceability, which enhances explainability and transparency to improve trust, and reduce the risk of poor-quality data creeping into strategic decision-making. Embedding trust into how an organisation captures, stores, uses and manages data is the starting point for what EY calls ‘Trusted Intelligence’.⁹
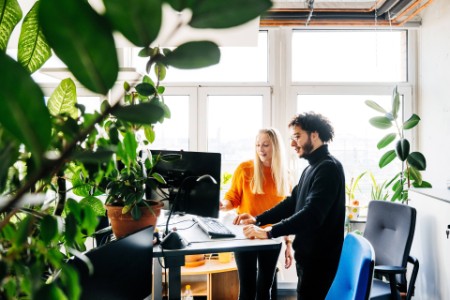
Chapter 2
Accessing data skills
Data skills have become a ‘must-have’ for all businesses. But relying solely on recruiting new talent won’t help fill the gap.
The availability of skilled resources to implement, maintain and improve data capabilities was identified by organisations in our survey as the most frequent and problematic barrier to the adoption and improvement of data foundations. Skills appeared to be lacking across a range of areas, including governance, architecture, analytics and cybersecurity.
Good talent with advanced skill sets in this space is pretty hard to find and demand is probably going to outstrip supply for a significant period.
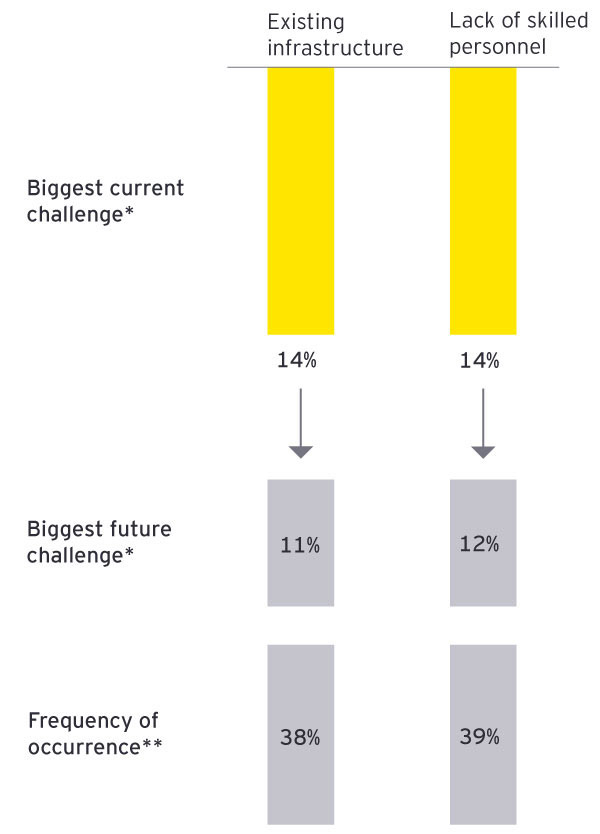
Current vs. future barriers and challenges to data foundations adoption
EY/DCMS
* Most problematic challenge currently experienced and expected in the future (within the next three years)
** Percentage of respondents who experienced challenge when improving organisation’s data foundations
Based on further interviews, we learned that the existing workforce in many businesses often had relatively poor data and digital skills, which meant that they could not use or analyse data effectively. These findings reinforce existing research by The Learning and Work Institute, published in March 2021, which showed that fewer than half of British employees are leaving full-time education with sufficient digital skills for the workplace, and three-quarters of employers reported that this was hitting their profitability.¹⁰
The problem of access to skills is made worse by the relative scarcity of experienced candidates to meet the massive demand. Many businesses we interviewed said that they needed to offer inflated rewards to attract and retain suitably talented people with data analysis and engineering, computer science, and AI skills – an affordability challenge for all organisations but a particularly acute problem for SMEs and the third sector.
A combination of these deep technical skills with broader commercial and entrepreneurial expertise is exceedingly rare. Indeed, analysis by the Royal Society showed that between 2013 and 2019 there was more than a doubling in the number of UK job-listings for “Data Scientists and Advanced Analysts” driven predominantly by the increased number of open vacancies for Data Scientists and Data Engineers.¹¹ Yet, our interviewees suggested that this was the ideal blend of skills that were needed to improve data foundations.
What can businesses do to improve access to data skills?
Data foundations and AI adoption in the UK private and third sectors, Ernst & Young LLP, July 2021
68%of the respondents to our survey believed that the Government has a role to play in helping organisations use data more effectively.
More than two-thirds (68%) of respondents believed that the Government has a role to play in helping organisations use data more effectively. Of those organisations considered by us to be AI ‘leaders’ – organisations who self-reported having strongly adopted AI in their businesses – 79% said that the Government’s main priority should be investment in developing relevant data skills.
Yet businesses have a crucial role to play, too. For example, the Institute for Apprenticeships lists 21 different apprenticeship standards involving data skills, including data analyst, data technician, data scientist, AI data specialist, and data protection and information governance practitioner, alongside a further 29 disciplines revolving around digital skills.¹² In 2020, EY welcomed a total of 854 graduates and 194 apprentices onto programmes including a tailored Tax Technology and Transformation apprenticeship and a four-year degree apprenticeship in Digital and Technology.¹³ These programmes provide opportunities for candidates, especially those from diverse and disadvantaged backgrounds, to work on real-life data and digital projects as permanent employees while simultaneously studying at leading academic institutions. Activating the apprenticeship levy provides a way for all businesses to access young talent and shape the skills needed to remain competitive.
As well as nurturing the data stars of tomorrow, the continued challenges with hiring for data skills are forcing businesses to seek alternatives, particularly through ‘upskilling’ – teaching existing employees additional skills – and reskilling – teaching new skills, especially to people who are unemployed or at risk of being made redundant by technology. The need to upskill and reskill existing staff in data and digital skills to cope with today’s frantic pace of technological change is well-documented. For example, The Future of Jobs Report 2020 by the World Economic Forum (WEF) suggests that around 40% of the global workforce will require reskilling by 2025.¹⁴
The Future of Jobs Report 2020
40%of the global workforce will require reskilling by 2025.
Again, within our own organisation, the EY Badges programme enables all employees to learn and earn digital badges for future-focused skills such as data visualisation, data science, AI and transformational intelligence.¹⁵
In the immediate term, as demand spikes and requirements become increasingly specialised, businesses can ensure that they build and maintain more flexible access to skilled individuals and freelancers. For example, the WEF reports that 41% of companies are seeking to expand their use of contractors, especially in such areas as Robotic Process Automation and AI.¹⁶
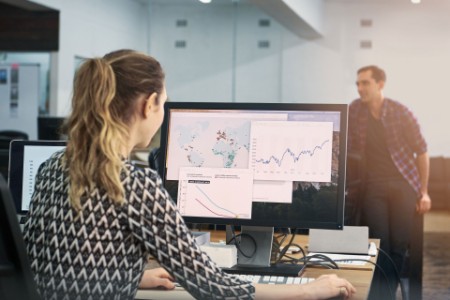
Chapter 3
Assessing the value of data
Despite appreciating that data is critical to future business success, most organisations struggle to measure returns on investment in data.
Our survey showed that almost all organisations, 99%, consider data to be at least moderately important to their success. Further in-depth interviews confirmed that data is a strategic priority for all organisations and around 80% believed that improving data foundations would deliver significant value through increased productivity. Other noteworthy benefits included increased revenue-generation (75% of respondents) and customer engagement (72%). Overall, 80% of respondents stated that the impact in these three areas alone would range from moderate to transformational.
Demonstrating value remains a challenge. There’s a lot of improvement required and only a finite resource. We need to demonstrate value to prioritise data foundations over and above delivery of other requirements.
Data foundations and AI adoption in the UK private and third sectors, Ernst & Young LLP, July 2021
99%of organisations surveyed consider data to be at least moderately important to their success.
Our research confirmed that strong data foundations, though necessary for underpinning many high-value business activities, are not considered sufficient to generate value directly. For example, investing in an enterprise data warehouse might make it easier for users across the business to access the data they need but, by itself, the warehouse has little intrinsic value. And, while businesses are aware that data quality improvements are essential, such foundation-building is often baked into wider organisational transformation programmes, which makes it challenging to allocate returns to data in general let alone to specific datasets. Overall, our research highlighted just how difficult it remains for businesses to close the gap between their optimistic forecasts of the value of data and the more mundane reality of data-related investments.
What can businesses do to assess the value of data more effectively?
For data and technology officers, the key to solving the data-value conundrum lies in assessing the value of outputs rather than inputs; instead of measuring the costs of improving data quality or accessibility, consider the net benefits of timely, detailed, insightful outcomes, products and processes.
For instance, during some interviews, we heard examples from leading organisations who were reducing the cost of manual data interventions, such as the time needed to find data, fix errors, or simply pass data from one system to another to improve automation. For businesses in fast-moving, customer-facing markets, such as consumer goods, retail, telecommunications or technology, improvements in data quality and accessibility were seen to allow more accurate and timely insights into customer behaviour. This data-driven customer-centricity was increasing the effectiveness of pricing decisions and improving customer retention and acquisition – all of which have clear and measurable business value.
When considering where to look for specific value-adding datasets, it can be helpful to consider that data, as many other digital assets, increases in value through interoperability. That is, combining different datasets can provide significantly more value to businesses than data used in individual siloes. The types of dataset that enable this kind of interoperability, and thus hold greater latent value, typically describe:
- Entities – including people, organisations, places and assets
- Events – including behaviour and actions that occur periodically, irregularly or at specific moments in time
- Locations – including points, addresses, boundaries and routes
The intersections between these three dimensions enable more sophisticated business decision-making, such as predicting churn by linking the characteristic behaviours of customers with events that take place at specific moments in time, recommending additional products to one customer based on the buying patterns of others, or predicting the future failure of a manufactured good based on shifting supply patterns in associated stock components.
Interoperability between datasets is both a necessary and often sufficient precursor to the use of machine learning – a branch of AI that uses software to learn patterns in data by being given lots of examples. Our research, though, suggested that only 37% of respondents, overall, considered AI to be a significant value-adding opportunity, although this varies considerably by sector and size of the organisation. For example, 52% of respondents in the finance industry said that their AI programmes would benefit from improvements in data foundations compared with only 18% from consumer products and retail. And 40% of large businesses saw the benefit compared with only 17% of SMEs.
An organisation’s ability to deploy AI successfully is itself a clear indication of the value of its underlying data, which hinges on quality and the remaining data foundations.
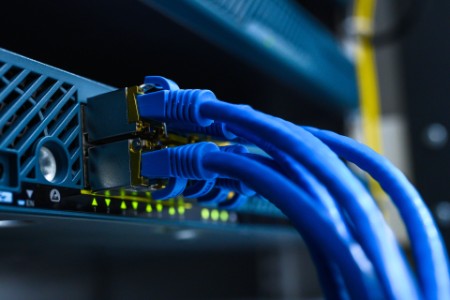
Chapter 4
Investing in technology and data infrastructure
The legacy systems and disparate technologies operated by some businesses simply aren’t good enough to meet modern data and AI requirements.
Alongside access to skills, our research demonstrated that challenges with existing infrastructure were also preventing many organisations from adopting and improving their data foundations.
We have a few legacy systems that work with our data and data processing. It is very tricky to persuade the business to dedicate resource to improving that ageing infrastructure.
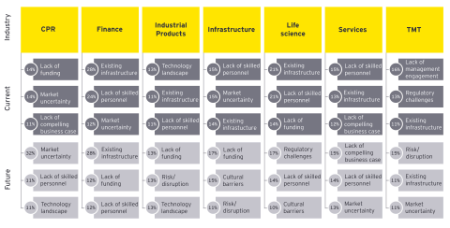
Top three barriers and challenges by industry (current and future)
EY/DCMS
Our research confirmed that the most common infrastructure issues are related to organisations operating disparate legacy technologies and data systems. Further interviews indicated that legacy systems were not always structured to facilitate advanced analytics or AI and will therefore require some additional level of future investment. These findings echoed those reported in a survey of companies conducted by Accounting Today in 2019, in which 92% of respondents said they planned to invest in data analytics in the subsequent year but only 28% believed their company had a suitable database to use analytics effectively.¹⁷
Not only do challenges with infrastructure make it more difficult to perform essential analytics but they also tend to stifle adoption of advanced technologies, such as AI. Our research showed that smaller businesses may be disproportionately affected. For instance, although around 90% of large organisations confirmed that they had already adopted AI or planned to do so soon, this dropped to less than 50% for SMEs.
This finding is consistent with our organisation’s experience in the UK: we’ve observed that AI is typically used by our clients to automate repetitive tasks, which tend to be more common in larger organisations because their size and complexity demand substantial process standardisation. Many SMEs, however, do not have the equivalent volume of standardised tasks and, therefore, will typically have fewer opportunities to embed AI. Even when they do identify an opportunity, it can take longer to accumulate sufficient benefits to offset the cost of AI initiatives.
What can businesses do to improve their technology and data infrastructure?
There are no shortcuts to improving data foundations if legacy infrastructure does not enable data to be used effectively. Even when companies invest heavily in the latest front-end technology and tools, if it’s not coupled with more flexible back-end infrastructure, the integrity of their data simply won’t improve.
Instead, as EY Americas Innovation Realized Leader Minsoo Pak says, “New cloud and infrastructure technology provides the architectural runway needed to support a rapidly evolving product roadmap.”¹⁸ As well as improving access to traditional workplace software for communication, collaboration and reporting, cloud technology also encompasses enterprise applications, such as enterprise resource planning (ERP) and customer relationship management (CRM). The key advantage as far as data is concerned is that a cloud-based approach – particularly when combined with a data fabric – simplifies application integration so that data can move around an organisation to be enriched, processed and visualised at any point of need rather than remaining locked up in legacy siloes or being replicated across multiple applications.
This kind of ‘platform thinking’ gives businesses an opportunity to consolidate their existing capabilities within a broader digital strategy refresh, and the following steps can help with this approach:¹⁹
- Define your vision, making sure that it takes account of your data-driven objectives
- Perform a cost-benefit analysis, considering both short- and long-term impacts within your business case
- Understand your current asset landscape, including base infrastructure, software and data, taking account of ongoing maintenance and enhancement needs as well as the anticipated complexity of any migration
- Look to improve your ways of working, remembering that migrating to the cloud does not automatically fix flawed processes
- Perform vendor due diligence, ensuring that their capability, support and cost models meet your requirements and constraints
- Redesign your resourcing model, ensuring that new programme-specific skills, such as project management cloud architecture and development, are introduced and roles associated with retired systems are redeployed
- Ensure your technology remains secure, accounting for both regulatory and third-party requirements for security, privacy and confidentiality of data
- Adopt cloud governance, ensuring appropriate accountability for and ownership of applications, data, security and other controls for cloud-based systems
- Begin operationalising immediately, rolling out training and change-management activities to help embed the new ways of working. Ensure that existing policies and procedures are reviewed and, where necessary, renewed for core areas of IT now covered by the cloud
Laying the right foundations
EY research for the DCMS points towards a sizeable gap between the potential and actual impact of data to drive both short-term economic and long-term social value in the UK. Despite evidence of ongoing investment and the consequential improvements being made in data foundations by businesses across all industry sectors, the gap remains unclosed because businesses continue to struggle with a range of data-related issues. These are most notably a lack of trust in their data, poor access to relevant skills, challenges with legacy infrastructure, and difficulties in identifying and measuring the value of their data. Until UK businesses can address these challenges, growth in emerging technologies, such as AI, 5G and the Internet of Things will also remain stunted.
It’s a fundamental truth that solid foundations are essential to the success of any future endeavour that relies upon data; it’s an inconvenient truth that improvements in data foundations alone are not sufficient to trigger success – the value can only be realised by using data to make more effective or more efficient decisions. This is a dilemma of sorts for most businesses, which must inevitably make judgements about how much investment is needed to improve data foundations without always having clear sight of where future value will come from.
EY research also highlights a divide between large businesses and SMEs (and the third sector). SMEs are more likely to be profoundly impacted by issues linked to data foundations. For example, they struggle more than large companies to access suitable technology and data infrastructure, and they can’t always compete in what is already an oversubscribed jobs market for data skills. This means that SMEs tend to lag larger companies in their adoption of AI and in unlocking the value of their data.
It’s clear that businesses, big or small, that are not able to improve their data foundations are likely to struggle to grow in an increasingly digital economy. For SMEs, especially, the challenge is acute. There are clear signs of optimism in our research: almost all businesses appreciated the importance of data to their success; almost all have dedicated data strategies or programmes of data improvement already in place; and almost all believed that improving data foundations would reap productivity benefits.
By drawing on technological approaches, such as data fabric, to improve the quality and consistency of use of their data, and turning to cloud-based infrastructure and services, businesses across all industry sectors can improve their data foundations. In addition, by focusing on the decisions and outcomes that lie at the heart of their strategies, businesses can more easily discover which datasets will lead to success and where they need to be made interoperable. Finally, although competing for talent on the open jobs market may be difficult, businesses can engage with established apprenticeship programmes and provide other opportunities for their existing workforces to upskill and reskill to ensure they have access to the skills the business needs in the future.
It is difficult to predict just how much value can be unlocked in the economy by improving data foundations. But our research suggests that businesses do understand the correlation between better use of data and increased economic and social value. The reality, though, is that they have little choice – no other resource, natural or artificial, provides the same degree of potential as data.
Summary
Getting the basics right in data, which means focusing on quality, skills, value, and infrastructure, contributes directly to successful digital transformations and more widespread adoption of trusted AI. These ‘data foundations’ are the key to long-term economic and social value.