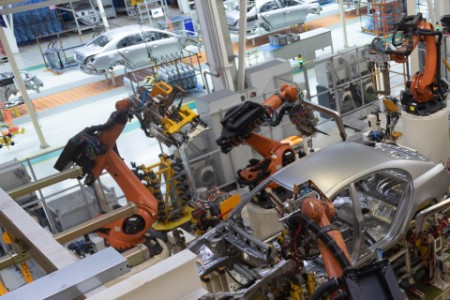
The better the question
How can AI in manufacturing help in aluminium production optimization?
The company sought to reduce energy cost by optimizing smelter operating parameters.
One of India’s largest integrated aluminium players was running its smelting operations on a smelter control technology, which was more than 40 years old. The age of the legacy control system and disconnected nature of the various Operating Technology (OT) and Information Technology (IT) platforms prevented a 360-degree visibility into existing processes and with desired granularity.
The decision making when it came to operations was largely driven by empirical rules and was not data driven. These empirical rules were not revised regularly.
The above resulted in large variations in energy consumption, production efficiency and process stability both within and across production lines. There was inadequate root cause analysis of events and the focus was primarily on post-facto analysis compared timely preventive and corrective action.
In order to arrest these inefficiencies, the company wanted to leverage data and analytics to transform their smelter operations. One of the specific areas in which it was looking to improve using data driven decision making was specific energy consumption of the primary aluminium production process.
The primary aluminium production is a closed loop electrolytic process (Hall-Heroult) and energy constitutes 60% of the overall production cost. The company wanted to explore analytics driven way of dynamically determining smelter operating parameters to maximize energy efficiency without sacrificing quality or productivity.
Related case study
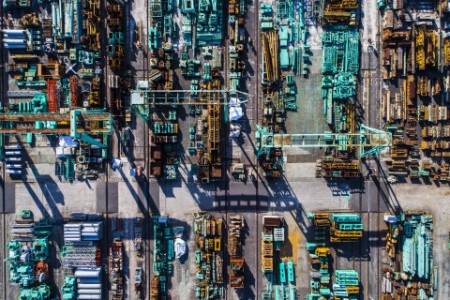
The better the answer
EY developed a Digital Twin to transform the company’s smelter operations
Our Digital Twin technology optimized operating parameters to drive energy cost reduction.
In order to address the company’s needs, EY constructed a comprehensive cloud-based Digital Twin solution for its smelter operations. The solution had four fundamental pillars.
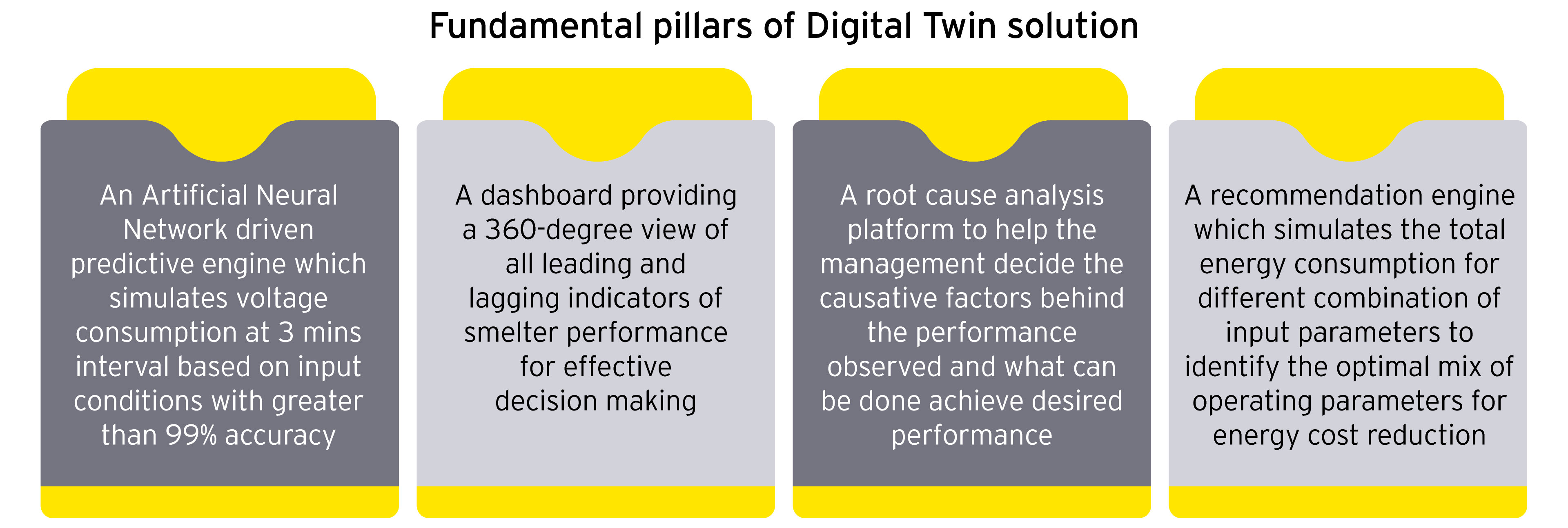
Source: EY’s digital transformation engagement team for an Indian aluminium producer
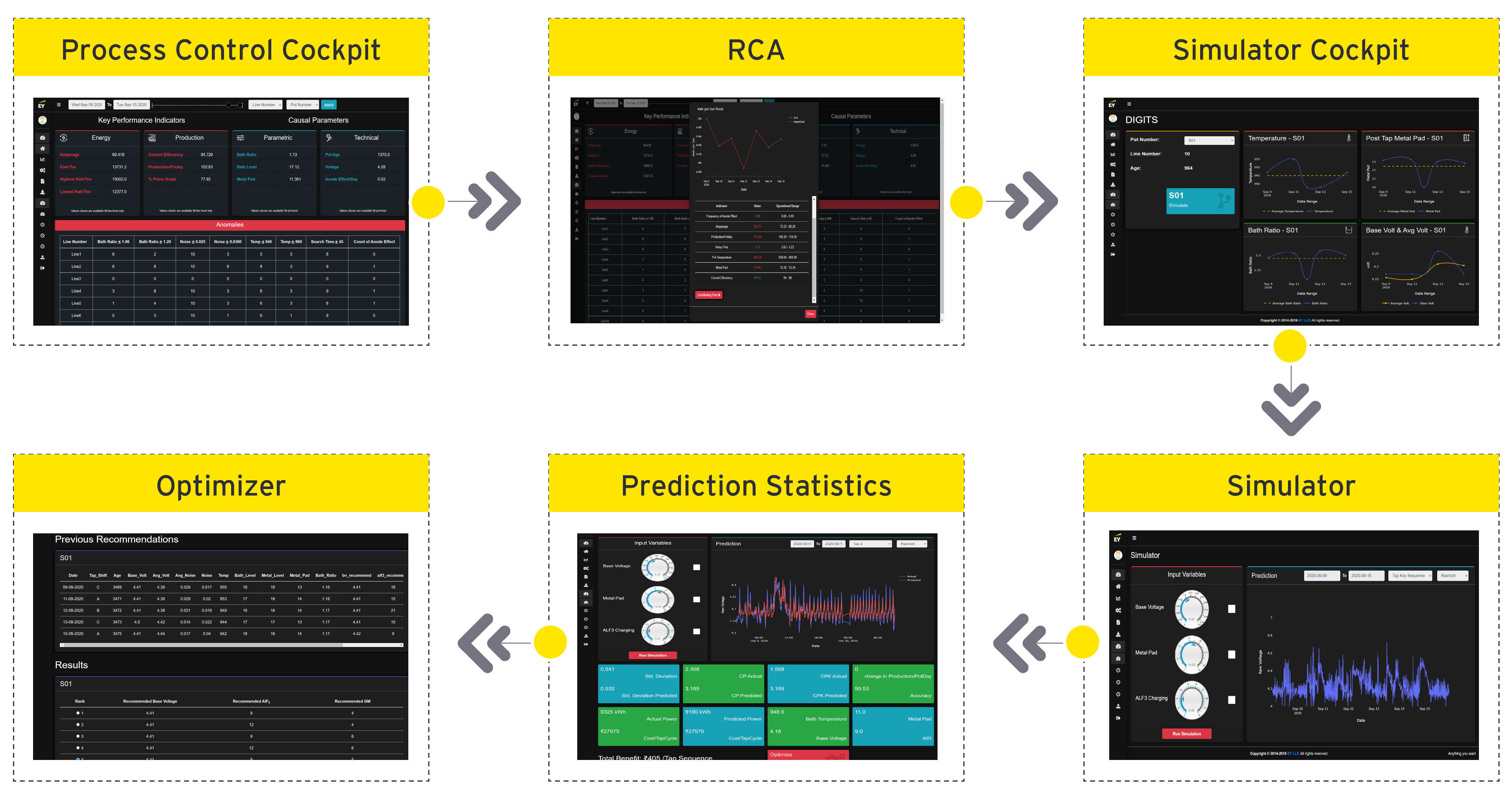
The solution provides shop-floor workers recommendations for each smelter pot on operating parameters such as the amount of base voltage to be provided to the pot, the amount of Aluminium Fluoride to be added and the amount of metal to be extracted at the time of metal extraction. The optimal selection of these operational parameters helps in reducing specific energy consumption of smelters without sacrificing process stability, quality and efficiency.
Today, the convergence of IT and OT along with the proliferation of cost-effective computing power and sophisticated AI/ML techniques allows us to push the frontiers of operational performance to levels deemed impossible even a decade back. I firmly believe that IIoT and Digital Twin combined are set to revolutionize manufacturing and usher in a new era of throughput improvement, quality control, and cost optimization.
An iterative solution development methodology was used and EY partnered with the company’s operations team to refine operating parameter recommendations to make the solution robust, scalable and error-free. The solution also creates detailed audit trail of all recommendations implemented to maintain traceability and tracking of outcomes so that underlying artificial intelligence models can become more intelligent over time.
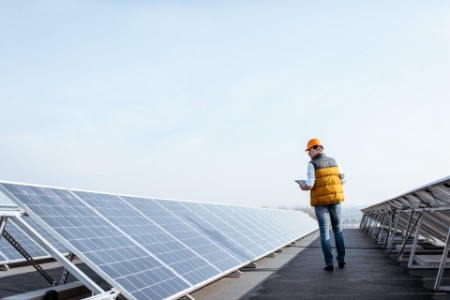
The better the world works
Use of Digital Twin resulted in 1.5% reduction in energy cost
Using Digital Twin, the client was able achieve the desired energy efficiency without sacrificing quality or productivity.
The solution provided the client an ability to have a comprehensive view of the current performance of smelter operation and the root cause driving the performance. This enabled the client to make more effective decisions and with the desired responsiveness.
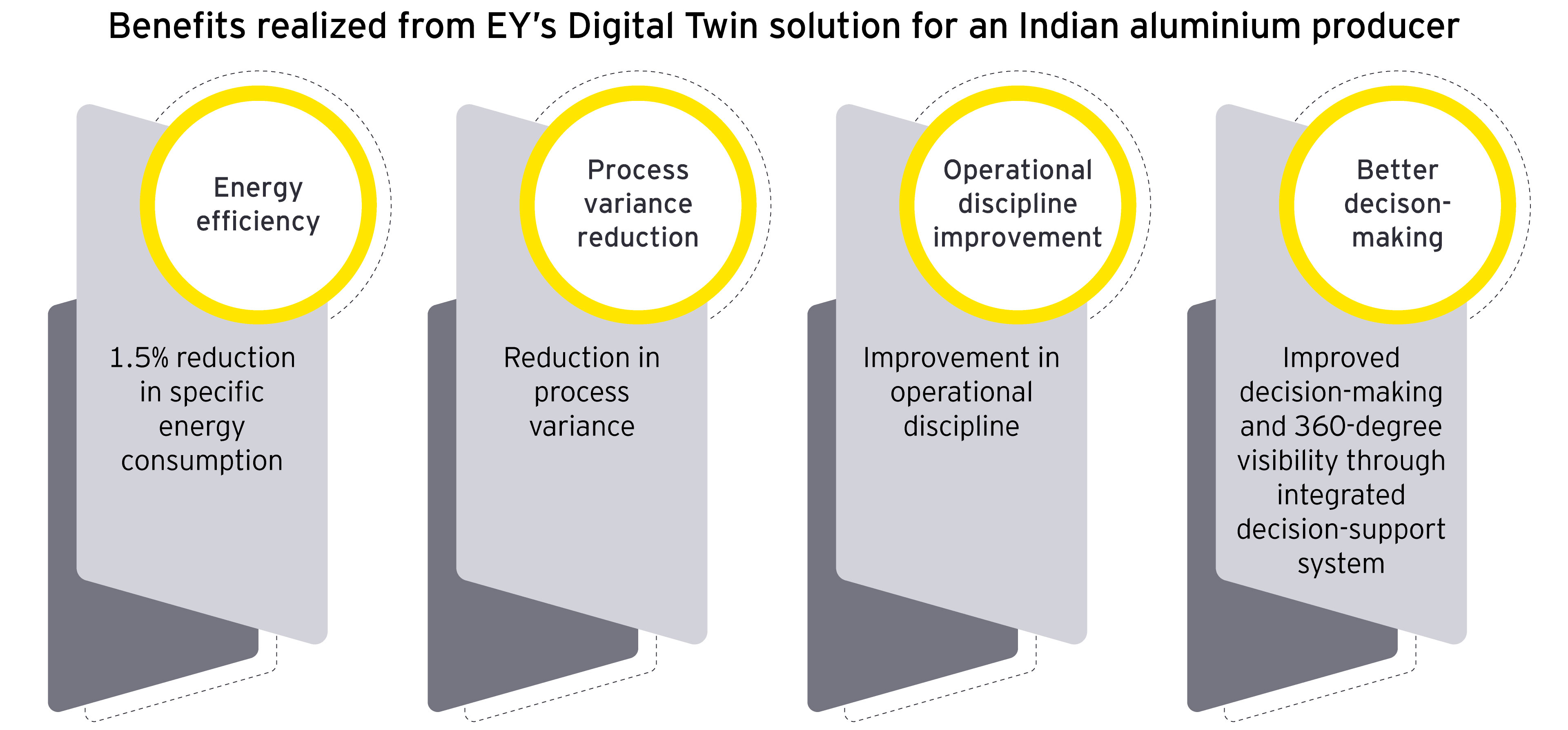
Source: EY’s digital transformation engagement team for an Indian aluminium producer
By using the operating parameters recommended by the Digital Twin, the client was able to unlock 1.5% reduction in annualized energy cost.
With the transition from experience driven decision making to data driven decision making, the solution helped the client in achieving consistency in operations across production lines.